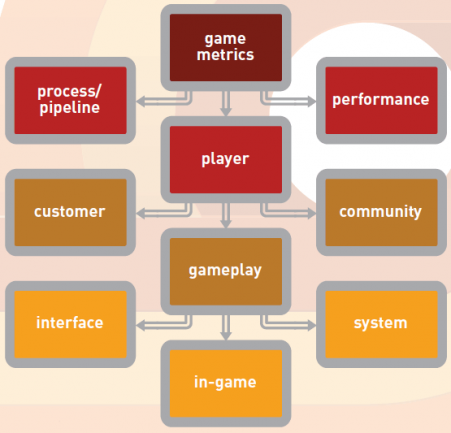
Counting customers in mobile business_The case of free to play
What: Prediction of users purchasing behavior in free to play models as it is a major challenge, the vital importance of managing marketing expenses and designing game mechanics.
Reason: measurement to increase the game’s profitability, identify their most profitable users.
Predict the potential value of customers is known as the customer lifetime value. One important aspect of CLV is to model the future purchases of a customer. The lifetime of gamer within days or frequent buyers tend to repurchase (user behavior) in a game is affected by the game mechanism.
Method: test the BG/NBD model (beta-geometric negative binomial distribution) for applicability in free to play
Data collection: the data tracking starts with the installation process of the game. A user is tracked with its own ID that related to any purchase that user does during their customer lifetime, including the amount spent (fixed price for a package) and the timestamp (day/month/year/hour/minute/second).
The data includes only customers that have at least made one purchase in the game. The lifetime of a customer is defined as the time between their first purchase and the last observable one.
They use several different measurements:
One will compare directly the repeat purchases per week for the real as well as the predicted data.
The predicted number of repeated transactions per customer can be used as an indicator of the model’s performance
Dataset: number of paying users, average lifetime, number of users with rep.purchases and the average number of purchases per user.
The model they use has a problem: underestimation in the beginning and an overestimation in the prediction period. The model is not able to reproduce the fast increase when the first period of time, a new customer is coming in and the number of purchase increase rapidly and the lifetime of a user is very short. However, at the end of the observed period, the user almost stopped purchasing while the model still expects repeat purchases.
Besides, the model underestimates the churned customers thus it overestimates the repeat purchase.
Therefore, the model will be difficult to deal with the fast increase and decline repeat purchases, especially with a short lifetime.
However, in a short lifetime game, the accuracy for churned customers is higher than the longer one. Because the time since the last purchase is the strongest indicator for the churn of customers.
In another side, in the longer lifetime game, prediction of heavy users works better and it is important because this cohort generates a major part of the revenue of the game.
Customer profitability and lifetime value
What: Segmentize highly committed customers who make repeat purchases and generate continual revenue streams also require minimal maintenance by tracking acquisition costs.
Reason: decide which customer to go after, how to change the promotional mix and may be discontinued serving specific customers
Method: determine customer profitability by analyzing relevant characteristics of customer activity with buying patterns of both new and existing customers. The firm distinguishes between segments of customers to respond similarly to a given level of communication/promotion: responsiveness of particular customers to certain offerings, the cost of making these offerings, the probability a customer is expected to keep generating revenue for the firm to enable to calculate of customer profitability and lifetime value.
Result: understanding the potential value of customers and prompting firms to learn more about the patterns of each customer, eliminate wasteful costs, and create a long-term perspective of the potential relationship with customers. Firms can tailor strategies to deal with different customer segments and customize different strategies for the same customer depending on the stage of the relationship between the customer and the firm.
Data: historical sales data, historical buying patterns to analyze the profits customer are likely to produce across their lifetime and future customer are predictable levels of loyalty.
Predicting player churn in the Wild
What: analyze and predict the behavior the behavior of the player is crucial to building a sustainable business
Purpose: detect leaving players which are expensive to recruit and incentivize them to remain in the game, maximize revenue. The model helps to emphasize on most important features around user engagement
Method: treat churn prediction as a binary classification task: churned or returning (churn/not -churn). The classifiers are trained on labeled data of already observed players but of course, require actual data of a target player for the prediction. Thus, given the information of a player up to a certain point in time, from now on referred to as cutoff date, the classifier decides if the player has churned or will return.
In the first and most straightforward interpretation of the churn prediction task, we consider a player without a single session after the cutoff date as churn. We will refer to this problem definition as P1. See Fig. 1(left) where these players are marked green while red is used for returning players. P1 only allows a very harsh decision on churning players, which is not very useful for real-world applications as with no play session left, the chances of reactivating the player in question is very low (at least from within the game). To relax this definition, and thus make it more applicable for real-world settings, we can label players as churners that have a low number of sessions or days to play after the cutoff date (soft churn). The remaining days of play have to fall into the range of a sliding window of size drlx. This relaxation can be interpreted as a separation of engaged and no longer engaged players, as opposed to the harsher separation of churned and non-churned players. From an industry point of view, this distinction is valuable as the players who are likely to quit playing soon are easier to reach and potentially incentivize to stay in the game, whereas players who have already left are harder to reach and incentivize. We will refer to this relaxed problem definition as P2. Fig. 1(right) shows an example of P2 and also allows for a comparison of P1 and P2.
Churned players as TRUE and returning players as FALSE. it is very important to decide on the absence time d(churn) (in days) after which a player is considered to have left the game.
Method 1: all churned players: positive examples.
To generate negative, they sample from all players that have played more than a single session, start with the first session and randomly select a cutoff session.
Method 2: take only sessions within a certain range into account. This approach takes a look at players on a chosen day and a time window of churn days.
Average Time Between Sessions now becomes the most important feature and appears in every decision tree
For M1, the features Number of Sessions and Number of Days occur most often. These two features were the most decisive features of all games.
Average Time Between Sessions was also observed frequently apart from game 1. This aligns well with the results reported in [13] where it was shown that the final intersession time is significantly longer than the early ones. an increase in the time between two sessions indicates that a player loses interest which is captured by the learned decision tree rules
d churn=7, because 95% percent of the absence times lie in this windows and it contains the full cycle of a week with every weekday
They also predicting churn is the proper selection of features that capture the behavior of the players
In-app purchases to free to play games: integrate 4 virtual economy related features: premium user flag, Predefined Spending Category, Number of Purchases and Average Spending per Session.
Why do people buy virtual goods? Attitude towards virtual good purchases versus game enjoyment.
Purchase behavior for virtual goods in free to play game environment
Hypotheses: enjoyment of the game reduces the willingness to buy virtual goods while at the same time it increases the willingness to play more of the game. Continued use, however, does positively predict purchase intentions for virtual goods.. (2) Attitude toward virtual goods and the beliefs about peers’ attitudes strongly increase the willingness to purchase virtual goods. Beyond these interesting results, the paper points to several further lines of inquiry.
Selling virtual goods entails problems of its own (only 2% of registered users convert into buying customers according to Forbes, 2013)
H1. Perceived enjoyment of the game is positively associated with continuous use intentions for the game.
H2. Perceived enjoyment of the game is negatively associated with purchase intentions for virtual goods. J. Hamari / International Journal of Information Management 35 (2015) 299–308 301
H3. Continuous use intention for the game is positively associated with purchase intention for virtual goods
One Response
I’m curious to find out what blog platform you are using? I’m experiencing some minor security issues with my latest website and I would like to find something more safeguarded. Do you have any suggestions?